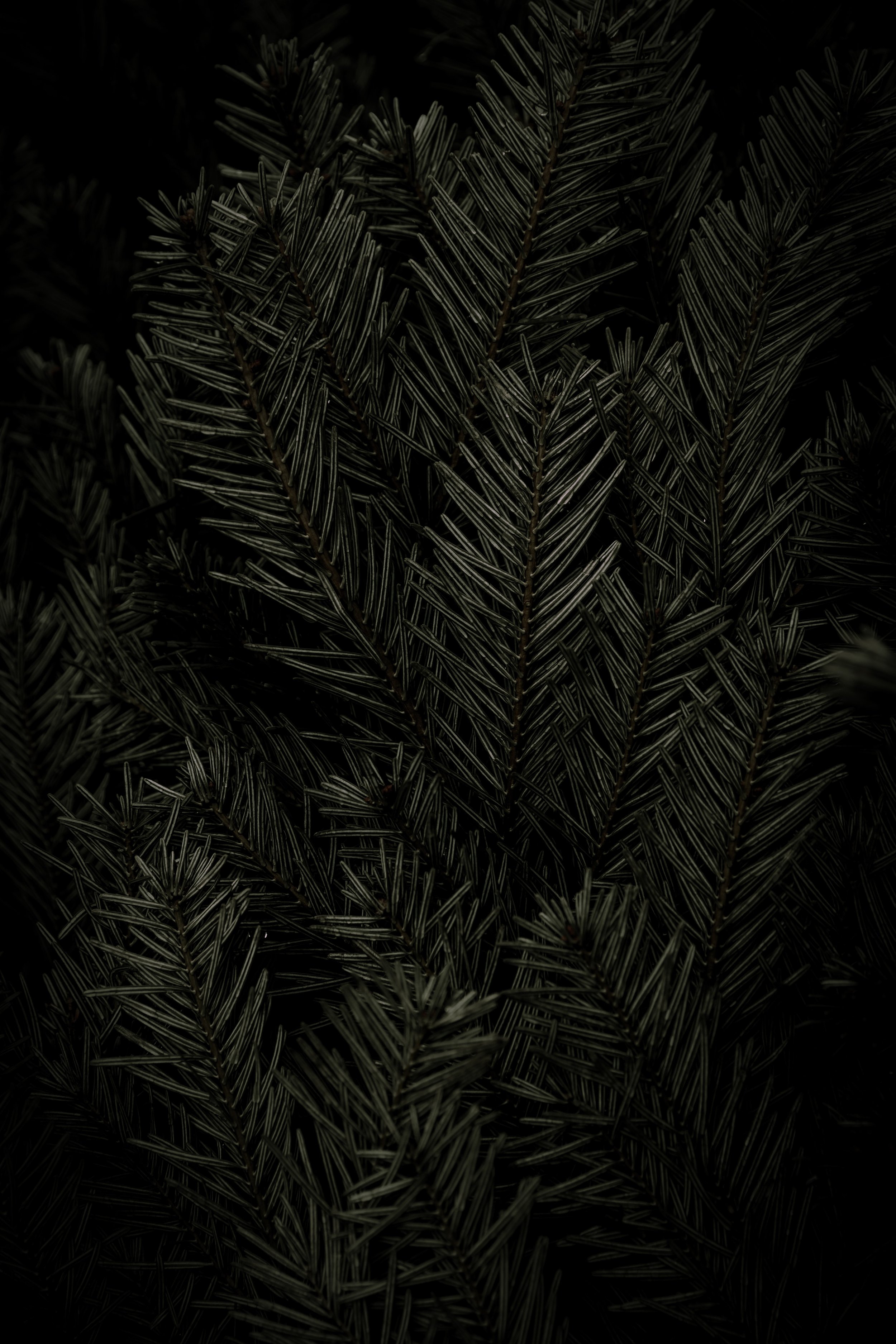
Better With Context
To show value for a messaging student engagement platform, we must be able to filter out the noise and identify important behavioral shifts.
Product Designer | Mainstay | November – December 2021
Project Deliverables
Research Plan
Research Analysis
Visual Design
Clickable Prototype
Annotated Designs
Skills and Experience
Research Participant Recruiting
Exploratory User Interviews
Usability Research
Visual Design
Prototyping
Data Visualization
About Mainstay
Mainstay is a student engagement platform that uses an AI chatbot to answer questions and nudge students towards great outcomes.
-
“At Mainstay, we believe one conversation can spark a brighter figure. Our engagement platform makes it easy for colleges and businesses to start and measure meaningful conversations that drive action at scale. From our rigorous research methods to our Behavioral Intelligence approach — everything we do is designed with our mission in mind: To help people take the next step toward a more fulfilling life.”
-
The AI communicates via SMS, web chat, Facebook chat, and Slack. Bots can provide university-specific answers 24/7, and automatically escalate urgent messages to a manager or advisor.
Outgoing messages and scripts can be scheduled to nudge contacts toward goals, reduce drop out rates in universities, reduce no show rates in businesses, and increase student and employee retention using regular touch points and sense of belonging.
-
Mainstay uses behavioral research to understand how to best nudge people towards their goals.
The research and writing teams work together to create content to inspire and direct users towards better engagement tactics.
Users
Bot Managers
The university staff members who write content, monitor conversations, schedule outreach messages, maintain bot knowledge, and/or pick up conversations when the bot can’t sufficiently answer.
Their Goals
Bot Managers want to support their students through school and encourage healthy habits (particularly important in the pandemic)
They want the best methods for keeping Contacts engaged.
They want to which students may have had too much or too little outreach so communication can be adjusted
Bot Managers use opt-out rates and messaging frequency to identify broad shifts in behavior and preference
Their Challenges
Need regular data updates because they walk a thin line between over- and under-communicating
Difficult to see the cause of a shift in behavior – only that there was a spike or dip in messages.
Student behavior is highly sensitive to outgoing campaigns, but campaigns are not identified in relevant graphs
Bot Managers know to look for all the information they need, but it is time consuming to find and analyze when there are more urgent messaging tasks
Contacts
The students who receive these outreach messages, respond to interactive messages, and/or ask the bot questions, all through SMS or Webchat.
Their Goals
Students aim to graduate on time and within their means
People don’t want to be bothered with unimportant, or poorly timed information
At the end of a semester, students have sent messages to their bot thanking the team for gentle nudges, timely support, and consistent encouragement.
Their Challenges
Today, more than 20% of students in undergraduate education are over the age of 24, leading busy lives with obligations such as children and ongoing careers
Modern students face a barrage of information, and can easily lose track of dates, deadlines, and important tasks
Students need to reserve their limited attention for life’s daily tasks
Business Problem
Bot Managers need regular updates about student behavior that can help them:
Identify messaging and topic trends
Measure the impact of their messaging
Validate the bot’s impact on student success
Bot managers found themselves struggling to identify at risk student groups or convince executives of the power of the platform.
Data-minded users who know what they are doing can create visualizations and find trends using raw CSV reports. The majority of our Bot Managers did not have the time or knowledge to do this on their own.
Process
The Mainstay design team works in three-week design sprints. Each designer completes all of the following every 1-2 sprints:
Gather information to understand the problem space
Brainstorm solutions with members of the product & development teams
Set learning goals which can validate–or redirect–the proposed solutions
Prototype the solution and write a mod guide
Recruit test participants from our existing users
Test the design, then analyze and share the results
Update final designs and/or re-assess the following sprint if necessary
Brainstorming
During the cross-functional sketching session, we stumbled upon a few solutions for this data blindness:
focus on groups of contacts, rather than an average of all contacts, to show broader trends
automatically identify anomalies that could be important indicators of at-risk student groups
Show possible causes for unusual trends close to the data to provide better context
Cross functional sketching sessions are a great chance to identify additional technical constraints and capture several divergent ideas.
First Prototype Draft
After the sketching workshop, we knew we needed a way to compare several groups against one another, maintain some of the existing data, and provide an easy way to find impactful insights about how students are responding to the chatbot.
Additional Iterations
In order to quickly identify outliers without any effort from Bot Managers, I recommended a selection of superlatives that could identify the most active or least active student groups.
A cross-functional call with the development team revealed that these data points ) would provide little insight and take minutes each page load.
The following iterations were designed to adjust for this issue.
Key Audiences initially started out as a pre-selected list of superlatives. In this prototype I maintained the same idea, but suggested a standard refresh time so we could gather new data during off-hours to compensate for the slow load times.
Interviews with Bot Managers showed that only some of the existing data visualizations were important to each person, and others were just noise. In this prototype, I combined the graphs using tabs as a way to keep that data available without overwhelming users.
To make sure this information was being offered in the right place, I also designed a version that shows this information directly on the audience details pages. Here, Key Audiences feature could work on demand using buttons at the top of the page. This could allow for more specific filters, and could have a faster load time.
Research
Each sprint, I personally recruited 3-5 test participants from our current users so the team could test designs with people who are familiar with the complex platform and bot management tasks.
Learning Goals and Key Takeaways
Is the data we're showing digestible?
Participants immediately understood the purpose and value of the Key Audiences summary.
They all rated the page as more approachable as compared to the way they find this data today.
Are the insights valuable?
Some participants mentioned creating visualizations like this on their own with raw data. These updates would save them time every week.
All participants found the combined graphs significantly more valuable.
Key Audiences highlighted important information participants said they would not have sought on their own.
Is the new data solution discoverable?
We were able to improve discoverability by including a few different locations for this information.
Allowing Bot Managers to save a filter set would make it easier for them to come back to a particular data comparison regularly.
Is the solution comprehensible?
Participants responded positively to the new data visualizations, and all three participants were able to find faster methods for getting to important insights.
With helpful default states and nudges in the UI, partners were able to compare groups of students and identify at-risk groups in the prototype.
Final Designs
This improvement to the insights page was more than exciting for users who wished to see the effect campaigns can have on key metrics.
An updated pattern for filtering allowed us to streamline other instances of filtering around the platform. Participants mentioned they would likely look at the same few filter sets often, so I provided a way to easily save a filter for later.
Downloading a chart to share with a colleague or manager was a regular task for participants, and many other bot managers have reported this in past interviews. I maintained a quick access download button for all graphs provided.
One major win for this project was an updated iteration of the timing graphs. By combining usage information, we were able to show when students engage throughout the week. Participants were excited to use this heat map to set more appropriate office hours according to student messaging tendencies.
Clear annotations are necessary for new visualizations. Here is an example of one of my figma files when I'm ready to hand off work to the development team. I made myself available for short calls and additional edge cases the engineers find as they begin to dig into development.
The final state for Key Audiences included actionable steps for student groups that may need more investigation or additional outreach.